Application of Geographically Weighted Regression for studying natural gas consumption and price elasticity from microdata
Conference
64th ISI World Statistics Congress - Ottawa, Canada
Format: CPS Abstract
Keywords: elasticity, gas, price
Session: CPS 04 - Finance and business statistics II
Monday 17 July 8:30 a.m. - 9:40 a.m. (Canada/Eastern)
Session: CPS 74 - Employment statistics
Wednesday 19 July 8:30 a.m. - 9:40 a.m. (Canada/Eastern)
Abstract
Natural gas consumption at the local level plays a pivotal role in total energy demand and it is widely utilized in many fields, such as homes (for heating, cooking and hot water), electricity generation, transportation, and manufacturing activities. At the local level, natural gas is distributed to end-users by local suppliers through low-pressure pipelines. Local distributors connect customers to the national gas grid, ensuring safety for consumers and measuring flow. The accurate study of spatial demand patterns is crucial for distributors, energy sellers and local authorities for several reasons: for the routine maintenance of networks and plants, for the optimum allocation of investments in territories, to analyze safety, to establish pricing and technical strategies, to manage an increase in energy demand, deal with dynamic demand and to obtain a cheaper supply of natural gas.
This paper seeks to take the literature one step forward by studying energy consumption from spatial microdata. Implementing spatial statistical methods to forecast natural gas consumption at the individual level can be helpful for energy suppliers. Geographic simulations of demand can significantly improve the technical and economic maintenance of supply plants (e.g., networks, pipelines, metering tools, etc.). However, the direct study of local energy consumption through spatio-temporal models is arduous and not always feasible for two main reasons. First, computational demand can be huge due to a large number of observations. Second, the low spatial variability in explanatory variables due to the local characterization of data and the lack of individual information (e.g., age of buildings, size of homes, type of heating system, number of residents, etc.) can lead to inconsistent outcomes. However, spatial information can be integrated with other regression techniques to overcome these drawbacks.
For example, in this paper, we show how the spatial location of end-users is useful in solving the endogeneity problem in the relationship between price and demand. Indeed, understanding end-user sensitivity to price changes provides essential insights into the impacts of policy decisions. For instance, the distributional consequences of taxation or subsidy policies depend on the price elasticity of demand. Therefore, the spatial patterns of price elasticity can reveal the areas that are influenced by policy decisions to a greater or lesser degree.
We developed and estimated several panel data models, trying to mitigate the endogeneity problem in the price-demand correlation. Moreover, we found that local macro-trends can be used as a proxy for individual information. The data were clustered through the postcode; however, the spatial dimension for individuals was ignored, in line with the existing literature.
This paper shows that spatial data can be a source of information to study price elasticity in energy consumption studies. Our analysis seeks to contribute to the previous literature, using the Geographically Weighted Regression (GWR) method, integrated with panel data models, to study energy consumption and price elasticity at the micro level. This spatial-regression method was chosen for its ability to regress locally varying instead of global coefficient as in the traditional OLS model.
Figures/Tables
comuni
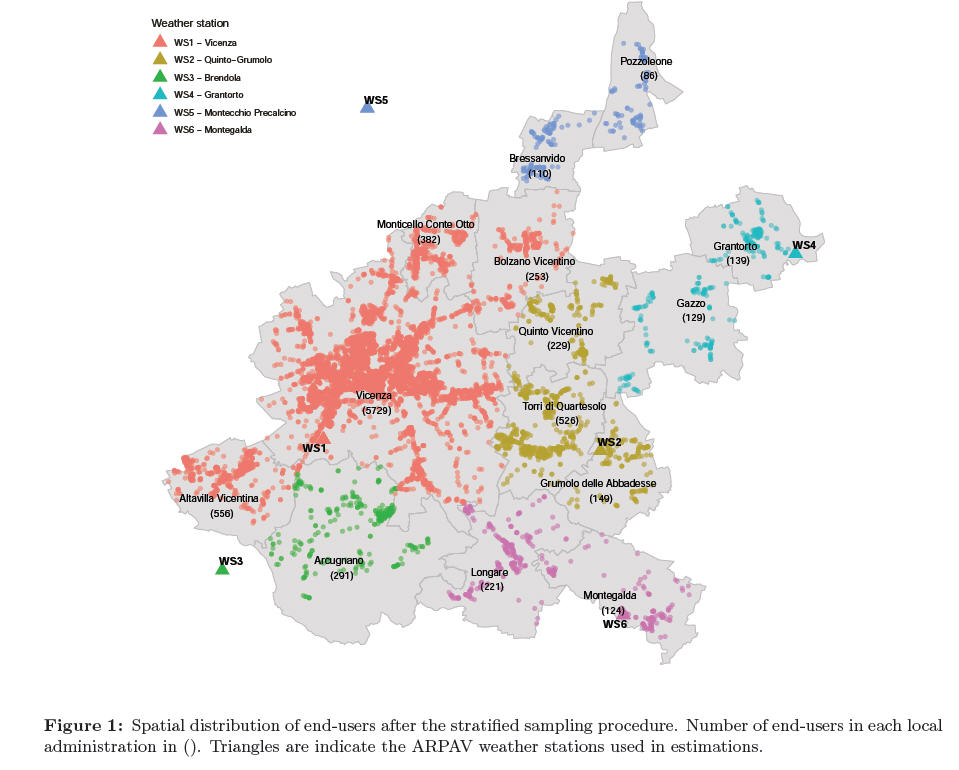
fig2
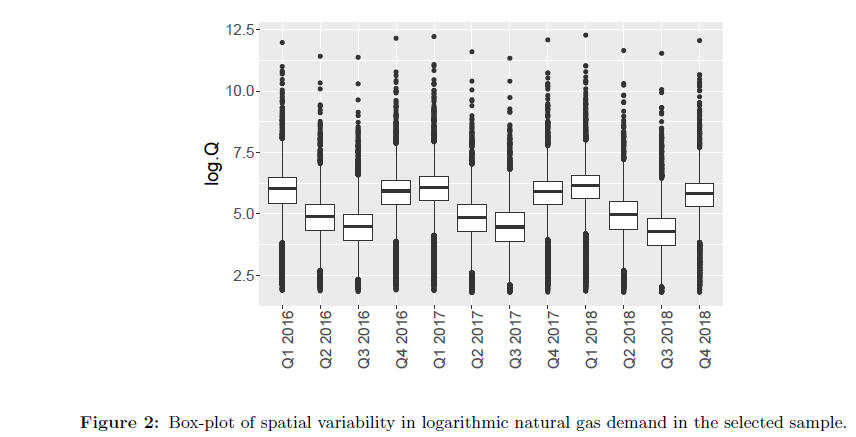
fig3
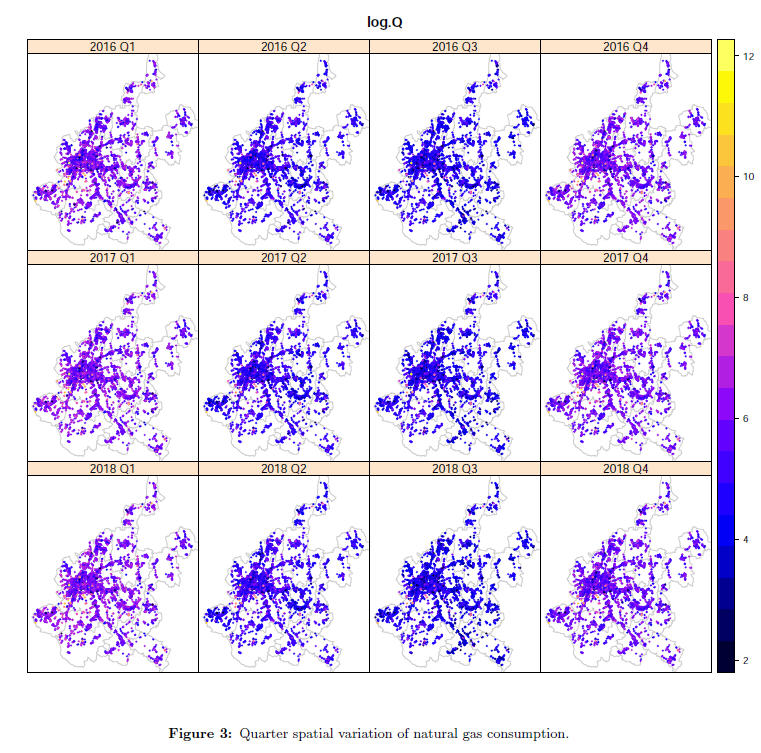
fig5
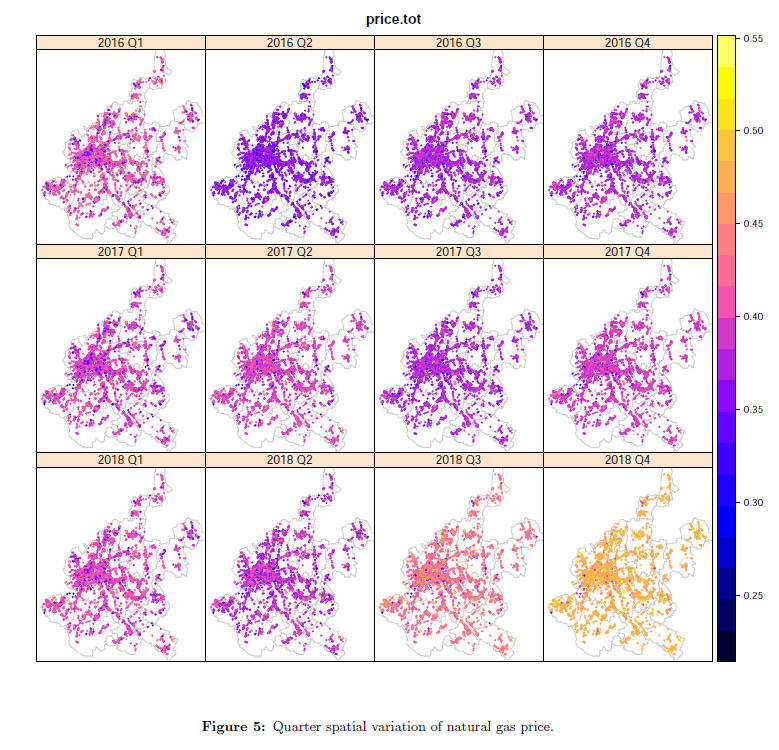
table3
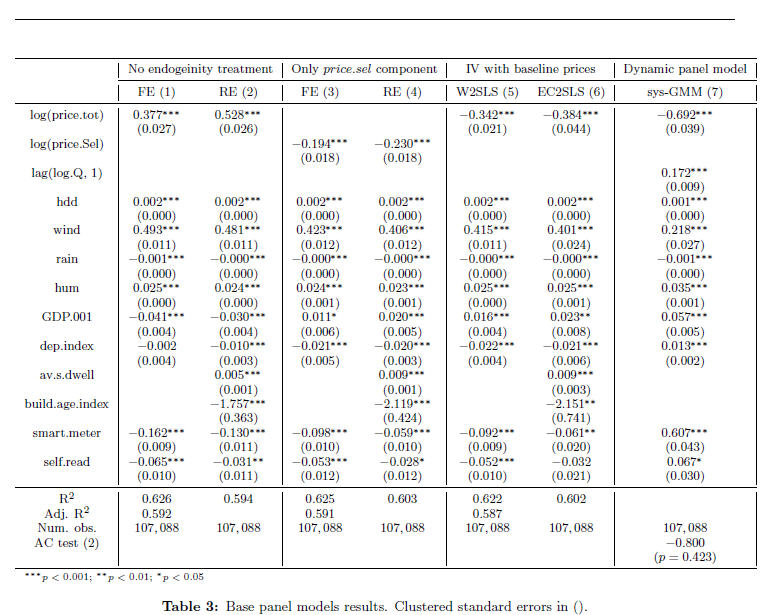
table4
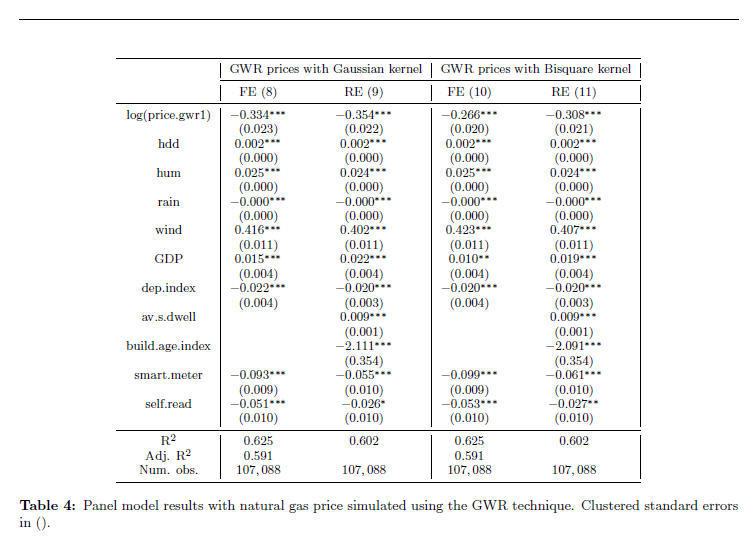