Non-parametric test for Markov Regime Switching Model with Intervention Parameter for Measuring Epileptic Seizure based on EEG
Conference
64th ISI World Statistics Congress - Ottawa, Canada
Format: CPS Abstract
Keywords: "bayesian, markov-chain, mcmc, non-parametric, time-series
Session: CPS 43 - Statistical modelling VII
Tuesday 18 July 8:30 a.m. - 9:40 a.m. (Canada/Eastern)
Abstract
In modeling electroencephalograms (EEG) of patients with epilepsy, one common interest is studying the magnitude of seizure on the brain signal. The volatility of these brain signals together with the presence of seizure makes the analysis more complicated. This paper proposed a method of modeling seizures in EEG by having a Markov hidden state process. We present a method of measuring magnitude of seizure that allows the regimes to be a function intervention parameters (step and pulse) at different time points. A simulation study was done to see the effect of intervention types, magnitude of intervention, and location of intervention on the power and size of the test. The study used Markov Chain Monte Carlo with backfitting algorithm to estimate the parameters and bootstrap approach for hypothesis testing. The simulation study indicated that the test is correctly sized and powerful when the intervention is of a pulse-type. When applied to one region of EEG, the model provided MAPE of 5.25% pre-seizure and 7.08% post-seizure. It also have shown that the distribution of state is more concentrated to high state when time of seizure is approaching.
Figures/Tables
FittedSeries
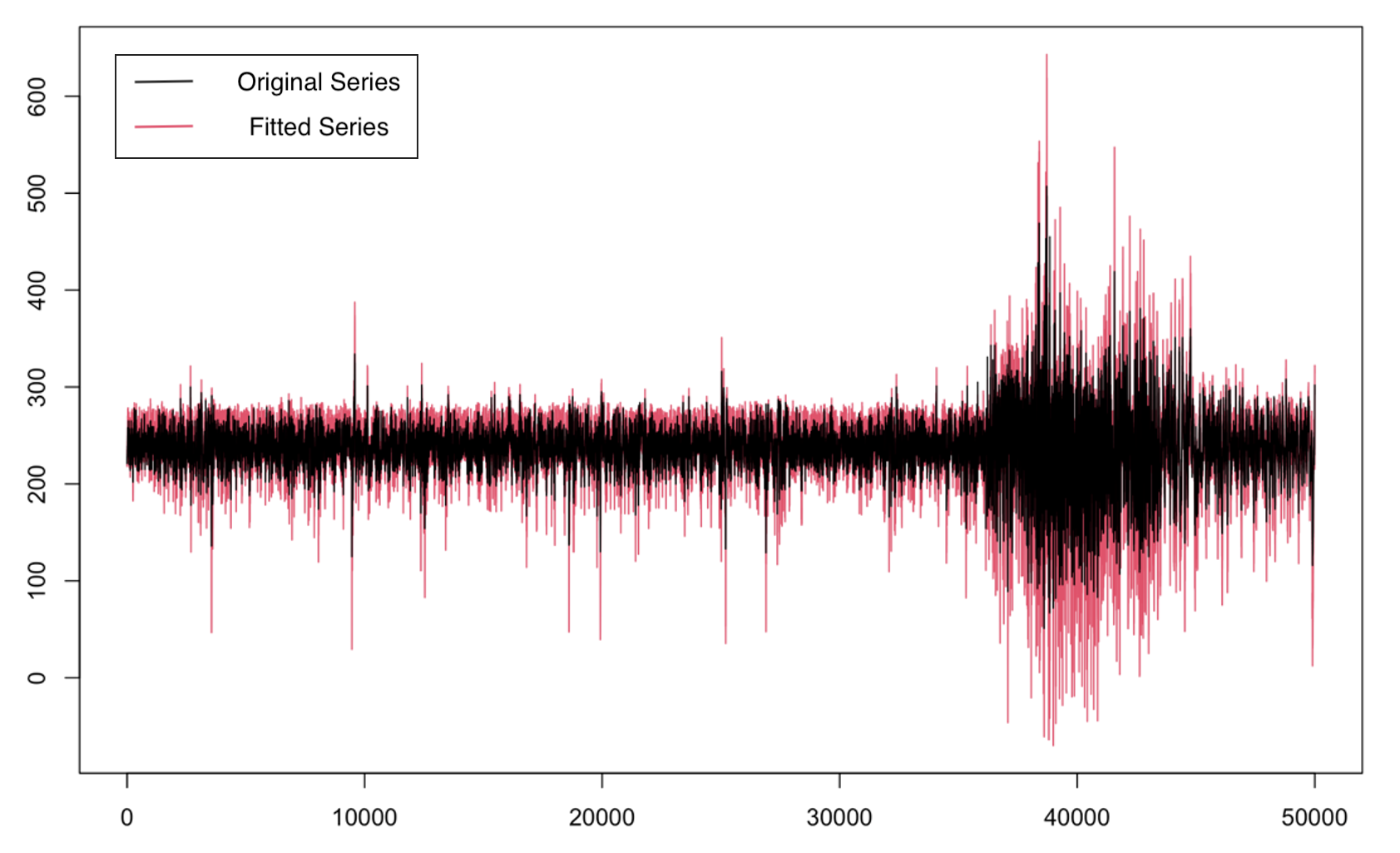